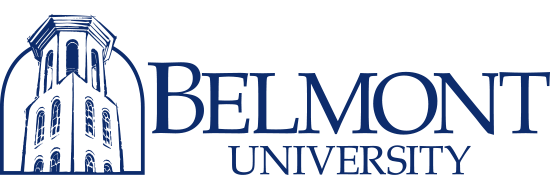
Belmont University Research Symposium (BURS)
Publication Date
Spring 2023
College
Sciences and Mathematics, College of
Department
Chemistry and Physics, Department of
BURS Faculty Advisor
Dr. Scott Hawley PhD.
Presentation Type
Oral Presentation
Abstract
Guitar players have been modifying their guitar tone with audio effects ever since the mid-20th century. Traditionally, these effects have been achieved by passing a guitar signal through a series of electronic circuits which modify the signal to produce the desired audio effect. With advances in computer technology, audio “plugins” have been created to produce audio effects digitally through programming algorithms. More recently, machine learning researchers have been exploring the use of neural networks to replicate and produce audio effects initially created by analog and digital effects units. Recurrent Neural Networks have proven to be exceptional at modeling audio effects such as overdrive, distortion, and compression. This research aims to analyze the inner workings of these neural networks and how they can replicate audio effects to such a high caliber. A Long Short-Term Memory (LSTM) Recurrent Neural Network (RNN) will also be used to model a distortion effect unit and compare the results they yield with the original audio device modeled.
Recommended Citation
Koch, Caleb; Hawley, Scott; and Fyfe, Andrew, "Investigating the Use of Recurrent Neural Networks in Modeling Guitar Distortion Effects" (2023). Belmont University Research Symposium (BURS). 197.
https://repository.belmont.edu/burs/197
Included in
Artificial Intelligence and Robotics Commons, Computer Engineering Commons, Data Science Commons, Music Commons, Signal Processing Commons, Software Engineering Commons, Theory and Algorithms Commons